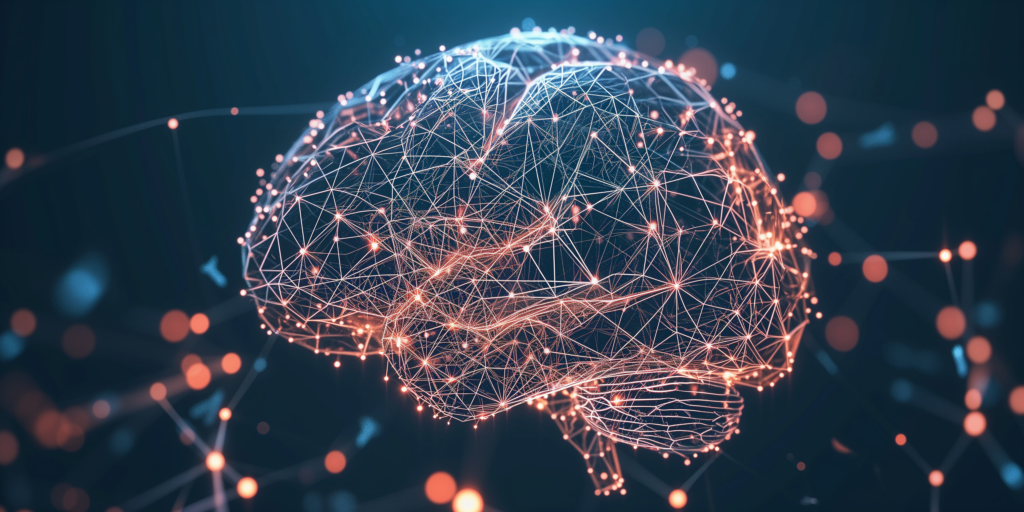
Deep learning, frequently discussed in AI, marks a significant advancement in machine capability. It’s a subset of AI inspired by the human brain’s structure and function. Deep learning uses hierarchical artificial neural networks for machine learning processes.
These networks mimic the human brain, with interconnected neuron nodes. Unlike traditional methods, deep learning enables nonlinear data processing. One of the most fascinating parts of deep learning is its capacity to handle vast amounts of data. Unlike traditional machine learning, deep learning algorithms are enhanced by more data exposure.
This feature makes deep learning crucial for big data applications, surpassing traditional processing techniques’ limits. Deep learning’s surge in popularity is influenced by enhanced computing power, data growth, and algorithm progress. It powers services like search engines, recommendation systems, and e-commerce support. Despite challenges, its capacity to autonomously learn from vast unstructured data makes it versatile.
Understanding the Basics of Deep Learning
At its essence, deep learning is a machine learning method that teaches computers to imitate human learning. In deep learning, a computer model learns to perform classification tasks directly from images, text, or sound. Such models can achieve state-of-the-art accuracy, sometimes exceeding human-level performance.
These models are trained using a large set of labeled data and neural network architectures containing many layers. The fundamental component of a deep learning model is the artificial neuron, mimicking the human brain’s neuron. Like a biological neuron, an artificial one processes inputs, adjusts weights and activates functions to produce an output. In image recognition, weights may prioritize edges or colors in specific image areas.
Deep learning models are trained with vast labeled data sets and neural network structures. These networks learn features from data without manual extraction. A prevalent type is convolutional neural networks (CNN or ConvNet), which combine learned features with input data. They use 2D convolutional layers, which are ideal for processing 2D data like images.
CNNs eliminate manual feature extraction, so you do not need to identify features used to classify images. CNN works by extracting features directly from images. The relevant features are not pre-trained; they are learned while the network trains on a collection of images.
This automated feature extraction makes deep learning models highly accurate for computer vision tasks such as object classification. CNNs learn to recognize patterns in the pixel values of images like the human brain recognizes patterns.
This automated feature extraction makes deep learning models highly accurate for computer vision tasks such as object classification. CNNs learn to recognize patterns in the pixel values of images like the human brain recognizes patterns.
The Importance of Deep Learning in Modern Computing
Deep learning is an important element of data science, which includes statistics and predictive modeling. It is extremely useful for large amounts of data and complex problems. As a tool, deep learning has the potential to propel high-level abstract data analysis forward. It is beneficial for performing tasks like image recognition, speech recognition, and natural language processing.
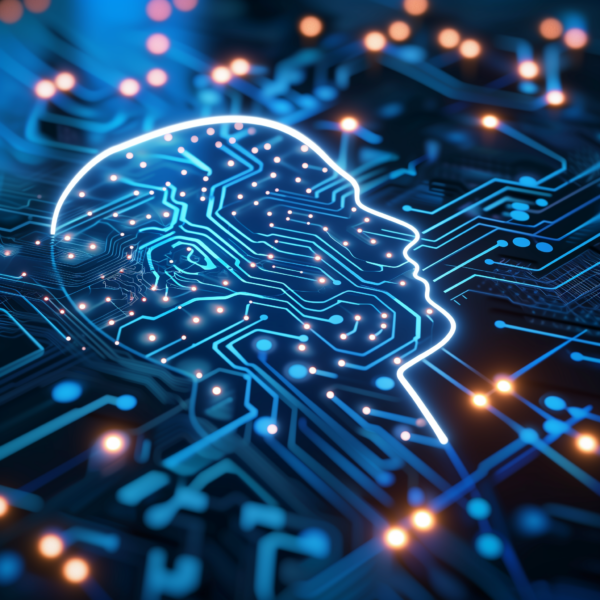
Today, deep learning is vital for tasks that are challenging for machines but simple for humans. Tasks like speech recognition, language translation, and decision-making rely on this technology. Deep learning enhances user experience by personalizing platforms.
While deep learning provides various advantages, it demands substantial computational power. The training procedure includes vast data and intricate algorithms requiring abundant computational resources.
This is where high-performance hardware, like GPU servers and specialized deep learning accelerators, becomes essential. These hardware solutions are designed to handle the massive parallel processing that deep learning tasks require.
Deep learning vs. Machine learning: In-depth Comparison
When discussing deep learning models and algorithms, grasping their place in machine learning is crucial. Though both are in AI, they differ in features and uses. Deep learning is linked to deep neural networks, which can learn independently from data. These networks imitate human decision-making, excelling at managing vast data volumes.
Machine learning, however, usually includes more classic algorithms needing structured and labeled data for training. Machine learning techniques depend more on human involvement to recognize features and offer essential training data. Conversely, deep learning techniques can automate tasks by recognizing and learning feature hierarchies from data, a notable advantage in fields like computer vision and natural language processing.
The methods in deep learning differ from traditional machine learning techniques. For example, deep learning algorithms create complex neural network architectures to recognize intricate patterns. This capability to manage complexity makes deep learning ideal for computer vision tasks like facial recognition. It’s also useful for processing data from audio, visual, and text sources.
Another key difference is the computing power required. Deep learning models often require more computational resources to train deep learning models, so cloud computing and dedicated graphics hardware use are more prevalent in deep learning. The reliance on cloud computing services and solutions allows for the necessary scale and flexibility in resources, enabling the deep learning process to be more efficient and cost-effective.
Key Differences Between Deep Learning and Machine Learning
The main distinctions between deep learning and machine learning are in their learning approach and task complexity. Deep learning excels in tasks like computer vision, speech recognition, and natural language processing by learning from vast data.
Machine learning, though potent, may need more human effort for feature development and suits simpler data and problems.
Deciding between deep learning and machine learning relies on the issue, data, and resources. Deep learning suits complex, unstructured data, while traditional methods work better for smaller, structured datasets.
Neural Networks Basics Explained
The foundation of deep learning is built upon artificial neural networks, which are algorithms modeled after the human brain. These deep-learning neural networks comprise interconnected nodes, or neurons, that work together to process and analyze data. Neural networks can learn from experience, improving their performance as they are exposed to more input data.
The deep neural network architectures are at the heart of these neural networks, which include multiple hidden layers between the input and output layers. These additional layers enable the network to learn complex patterns and data representations. This process is essential for tasks such as image and speech recognition.
Components of a Neural Network
A typical neural network consists of an input layer, one or more hidden layers, and an output layer. Each layer comprises units, or neurons, with weighted connections to neurons in the subsequent layer.
The weights of these connections are adjusted during the training process as the network learns from the data.
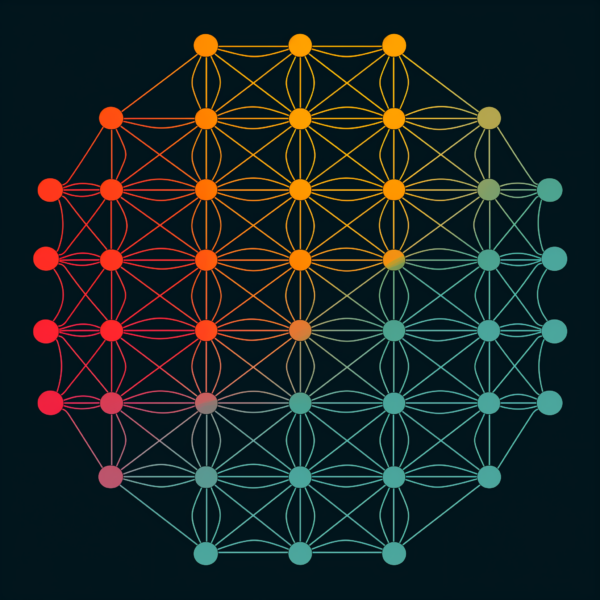
How Neural Networks Learn
Neural networks learn through a process called backpropagation, which involves adjusting the weights of the network connections based on the output error compared to the expected result.
This iterative learning process requires large amounts of data to train deep learning models effectively. Cloud computing nodes and dedicated graphics hardware can significantly speed up this process by providing the necessary computing power to handle the complex calculations involved in training.
In essence, grasping neural networks’ fundamentals and distinguishing deep learning from machine learning is vital for AI and ML experts. These tools drive complex applications and hint at AI and cloud computing’s future. Also, high-performance GPU servers for machine learning and cloud services access will be vital for maximizing deep learning methods’ capabilities.
Types of Neural Networks
Within deep learning, there are various types of neural networks, each designed with specific architectures to perform different tasks. Understanding these types is crucial for professionals who rely on deep learning methods, such as AI developers and machine learning professionals.
It allows them to select the most appropriate neural network architectures for their projects.
Convolutional Neural Networks (CNNs)
Convolutional neural networks, or CNNs, are a type of deep neural network specifically designed for processing data with a grid-like topology, such as images. CNNs are particularly powerful in computer vision, where they can identify and make sense of visual data with minimal human intervention.
These networks use a technique known as convolution, which allows them to filter inputs for helpful information and reduce the dimensions of the data, making it more manageable for processing.
Recurrent Neural Networks (RNNs)
Recurrent neural networks (RNNs) are another type of neural network best suited for sequential data such as time series, speech, or text. Unlike CNNs, RNNs have the unique feature of feeding information back into the network, which allows them to maintain a sort of ‘memory’ of previous inputs.
This characteristic makes RNNs ideal for tasks that require understanding context over time, such as language translation or speech recognition.
Hardware requirements For Deep Learning
The hardware requirements for deep learning are significant, as training deep learning models involves complex algorithms and large amounts of data.
In this section, we will explore the hardware components that enable the deep learning process, focusing on the needs of professionals who require GPU servers for their compute-intensive tasks.
GPUs and Their Role in Deep Learning
Graphics processing units (GPUs) are preferred for deep learning as they excel in parallel operations. This results in faster processing than traditional CPUs, especially for matrix and vector computations.
GPU servers can significantly reduce training time for deep learning models, benefiting professionals with deep learning skills. Selecting the right hardware is critical for efficiently running deep learning models. Factors to consider include the size and complexity of the model, the volume of data, and the expected training time.
Cloud-based GPU servers offer a flexible and scalable solution, allowing users to access high-performance computing resources without the upfront investment in physical hardware.
Pros and Cons of Using Deep Learning
While deep learning offers a range of benefits, it is also important to consider the pros and cons of using deep learning. This balanced perspective ensures that professionals can make informed decisions about when and how to apply deep learning techniques in their work.
Let’s see some of the benefits below:
- Deep learning includes its ability to automate tasks that would be difficult or impossible for humans to perform, such as analyzing large amounts of data for complex patterns.
- Deep learning models can also improve over time as they are exposed to more data, leading to increased accuracy in tasks like facial recognition and computer vision.
Despite its strengths, there are limitations to deep learning. One of the main challenges is the need for large amounts of labeled data to train deep learning models. Additionally, the computing power required for training can be substantial, leading to higher costs and potential environmental impacts due to energy consumption.
Deep Learning Use Cases
In the next lines, we will highlight some of the most compelling deep learning use cases.
Below, you will find two examples of how deep learning is used to solve real-world problems:
- Deep learning in healthcare: In healthcare, deep learning is leveraged to improve diagnostic accuracy, personalize treatment plans, and predict patient outcomes. By analyzing large amounts of data, deep learning models can identify patterns that may not be visible to human doctors, leading to earlier and more accurate diagnoses.
- Deep learning in autonomous vehicles: Autonomous vehicles’ development relies heavily on deep learning, particularly deep neural networks that can process and interpret large amounts of sensory data required for safe navigation. These techniques enable vehicles to recognize objects, make decisions, and learn from experience without human intervention.
As you can see, deep learning has various applications across various industries, demonstrating its versatility and power.
Challenges and Limitations
Despite deep learning’s promise, some challenges and limitations must be acknowledged. This section will address some key issues facing deep learning, from ethical considerations to technical hurdles.
Let’s see two examples below:
- Data privacy and security issues: As deep learning often involves handling sensitive information, data privacy and security are significant concerns. Ensuring personal data protection while training deep learning models is a complex challenge that requires robust security measures.
- Computational costs and environmental impact: The computational costs associated with deep learning are more than just financial. Running large-scale deep learning algorithms on GPU servers has a significant environmental impact, as it requires great amounts of energy, contributing to the technology’s carbon footprint.
While deep learning has revolutionized various industries, there are still some factors that need to be considered before fully implementing such services in your business.
Future Trends in Deep Learning
Looking ahead, the future trends in deep learning are poised to revolutionize technology further and how we interact with it.
Let’s see some of the emerging trends and what they could mean for deep learning:
- Emerging deep learning technologies: One of the most exciting future trends in deep learning is the development of new neural network architectures that push the boundaries of efficiency and performance. These advancements are expected to make deep learning models even more powerful and accessible, enabling them to solve increasingly complex problems more accurately.
- Deep learning and the evolution of AI: Integrating deep learning with other AI technologies leads to the creation of more sophisticated and capable AI systems. This synergy is expected to drive innovation across various sectors, transforming our lives and work by automating complex tasks and providing deeper insights into large amounts of data.
As deep learning continues to mature, we can anticipate a greater emphasis on transparency and explainability in AI models and advancements in unsupervised and reinforcement learning. Moreover, the convergence of deep learning with cloud computing and edge computing will likely enhance the scalability and efficiency of AI applications, making them more widely available across different platforms and devices.
Because of this, many GPU server providers such as MaxCloudON emerged, which allowed small and medium-sized enterprises or even individuals to have access to normally very expensive GPU servers.
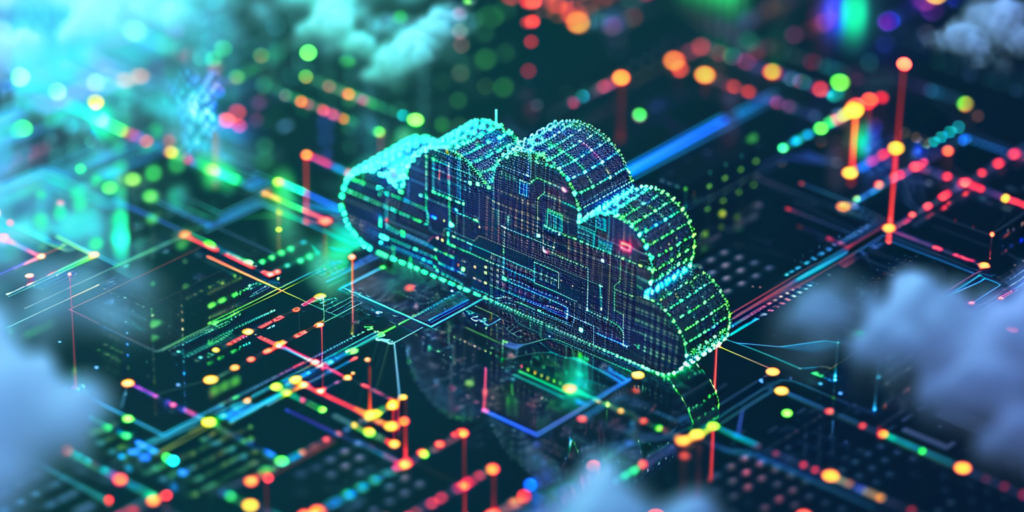
In conclusion, the future trends in deep learning indicate that technology is growing more advanced and more integrated into the fabric of various industries. As deep learning becomes more intertwined with cloud computing and AI, its potential to revolutionize different sectors of the economy is boundless.
Sources:
- NVIDIA – What is Deep Learning?
- DeepMind – Learning Resources
- IBM Cloud – Deep Learning
- Google AI Research
- Amazon Web Services – AI Services
Frequently Asked Questions:
-
How does cloud computing enhance deep learning capabilities?
Cloud computing provides scalable and flexible computing power essential for training deep learning models, especially those that require handling large datasets and performing complex computations. Cloud platforms like MaxCloudON provide on-demand access to dedicated GPUs for faster and more efficient model training without significant upfront investments in physical infrastructure.
-
How do I run my deep learning model on the cloud?
To run a deep learning model on the cloud, typically follow these steps:
1. Select a Cloud Service Provider: Choose one with GPUs and scalable infrastructure. Providers like MaxCloudON specialize in high-performance computing for deep learning.
2. Set Up a Cloud Environment: Create a cloud instance suited to your model’s needs, focusing on GPU or high-memory options.
3. Upload Data and Model: Move your datasets and model files to the cloud.
4. Install Software Tools: Install all necessary deep learning frameworks like TensorFlow, PyTorch, or Keras.
5. Configure Resources: Optimize CPU, GPU, and memory settings to meet your training requirements.
6. Train and Deploy: Run training scripts and deploy the model on the cloud for testing or production.MaxCloudON offers dynamic scaling and detailed guidance to streamline this process.
-
Which cloud is best for deep learning?
The best cloud for deep learning often depends on your specific needs. Consider the scale of your data, required processing power, budget, and preferred tools. Major providers like Amazon Web Services (AWS), Google Cloud Platform (GCP), and Microsoft Azure are popular because they offer robust, scalable environments with extensive machine learning services and GPU support. MaxCloudON is an attractive option for those prioritizing performance and cost when running deep learning models.